Notes on CS344: Introduction to Parallel Programming
Overview of CUDA processing pipeline:
1.Allocate host/device buffers, fill device buffers with data to process.
int *h_buffer = new int[num_elements];
memset(h_buffer, 0, num_elements * sizeof(int));
int *d_buffer_in;
cudaMalloc(&d_buffer_in, num_elements * sizeof(int));
cudaMemcpy(d_buffer_in, h_buffer, num_elements * sizeof(int), cudaMemcpyHostToDevice);
int *d_buffer_out;
cudaMalloc(&d_buffer_out, num_elements * sizeof(int));
2.Set parameters for kernel execution (grid size, thread block size, number of shared memory to allocate).
const dim3 blockSize(512, 1, 1); // specify 512 threads for a thread block
const dim3 gridSize(ceil(num_elements / blockSize.x), 1, 1); // will launch ceil(num_elements / blockSize.x) number of thread blocks
3.Launch a kernel with prepared data.
kernel<<<gridSize, blockSize>>>(d_buffer_in, d_buffer_out, num_elements);
If kernel uses Shared Memory, we can specify the amount of memory to allocate dynamically:
kernel<<<gridSize, blockSize, number_of_elements_in_shared_mem * sizeof(int)>>>(d_buffer_in, d_buffer_out, num_elements);
4.Synchronize CUDA device before retreiving data from device buffers.
cudaDeviceSynchronize();
Problem set #1: Color to Greyscale Conversion
RGB -> Grayscale conversion:
gray = .299f * R + .587f * G + .114f * B
Kernel implementation:
__global__
void rgba_to_greyscale(const uchar4* const rgbaImage, unsigned char* const greyImage, int numRows, int numCols)
{
//First create a mapping from the 2D block and grid locations
//to an absolute 2D location in the image, then use that to
//calculate a 1D offset
uint tidx = blockIdx.x * blockDim.x + threadIdx.x;
uint tidy = blockIdx.y * blockDim.y + threadIdx.y;
uint pos_1d = numCols * tidy + tidx;
if ( (tidx < numCols) && (tidy < numRows) )
{
float r = (float)rgbaImage[pos_1d].x;
float g = (float)rgbaImage[pos_1d].y;
float b = (float)rgbaImage[pos_1d].z;
float channelSum = __fadd_rn(__fadd_rn(__fmul_rn(.299f , r) , __fmul_rn(.587f , g)) , __fmul_rn(.114f , b));
greyImage[pos_1d] = static_cast<unsigned char>(channelSum);
}
}
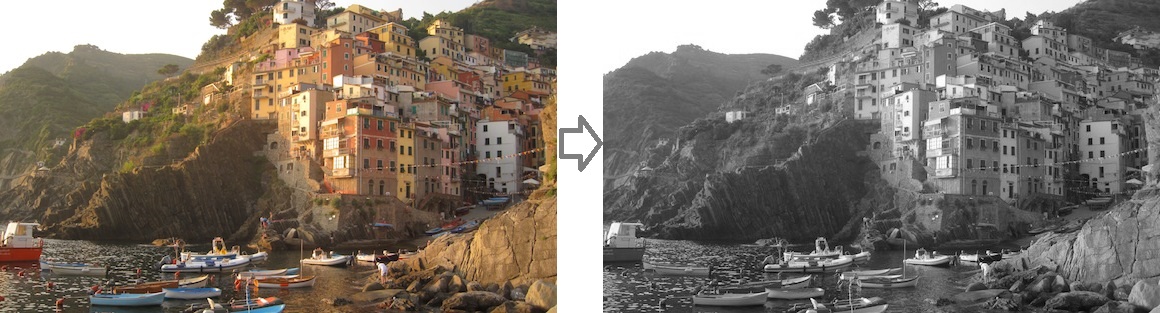
It’s interesting to notice that (X * Y) + Z calculated on CPU doesn’t equal (X * Y) + Z calculated on GPU, because GPU uses Fused Multiply-Add operation (FMA). So CPU version looks like Round(Round(X * Y) + Z)
and GPU with FMA Round(X * Y + Z)
. This moment may result in small difference between CPU and GPU output. In the kernel above I use explicit rounding to match the reference (CPU) result.
CPU: ~2.25 msec (Intel i3-6006U 2GHz)
GPU: ~0.67 msec (Nvidia 920MX)
Github link.
Problem set #2: Image Blurring
Input image is in RGB format in which color components are interleaved (RGBRGBRGBRGB…). Working with such structure will result in ineficient memory access pattern.
Steps to follow:
1.Separate RGB channels that different color components are stored contiguously (RRRRR…, GGGGG…, BBBBB…).
__global__
void separateChannels(const uchar4* const inputImageRGBA,
int numRows, int numCols,
unsigned char* const redChannel,
unsigned char* const greenChannel,
unsigned char* const blueChannel)
{
uint idx = blockIdx.x * blockDim.x + threadIdx.x;
uint idy = blockIdx.y * blockDim.y + threadIdx.y;
if ( idx >= numCols || idy >= numRows )
{
return;
}
uchar4 pixel = inputImageRGBA[idy * numCols + idx];
redChannel[idy * numCols + idx] = pixel.x;
greenChannel[idy * numCols + idx] = pixel.y;
blueChannel[idy * numCols + idx] = pixel.z;
}
2.Perform bluring on each color channel (convolve each channel with a filter).
__global__
void gaussian_blur(const unsigned char* const inputChannel,
unsigned char* const outputChannel,
int numRows, int numCols,
const float* const filter, const int filterWidth)
{
// NOTE: If a thread's absolute position 2D position is within the image, but some of
// its neighbors are outside the image, then you will need to be extra careful. Instead
// of trying to read such a neighbor value from GPU memory (which won't work because
// the value is out of bounds), you should explicitly clamp the neighbor values you read
// to be within the bounds of the image. If this is not clear to you, then please refer
// to sequential reference solution for the exact clamping semantics you should follow.
uint idx = blockIdx.x * blockDim.x + threadIdx.x;
uint idy = blockIdx.y * blockDim.y + threadIdx.y;
if ( idx >= numCols || idy >= numRows )
{
return;
}
float sum = 0.0;
for (int filter_y = -filterWidth/2; filter_y <= filterWidth/2; ++filter_y) {
for (int filter_x = -filterWidth/2; filter_x <= filterWidth/2; ++filter_x) {
int image_vert_clamp = MIN(MAX(filter_y + idy, 0), static_cast<int>(numRows - 1));
int image_horiz_clamp = MIN(MAX(filter_x + idx, 0), static_cast<int>(numCols - 1));
float img_value = static_cast<float>(inputChannel[image_vert_clamp * numCols + image_horiz_clamp]);
float filter_value = filter[(filterWidth/2 + filter_y)*filterWidth + (filterWidth/2 + filter_x)];
sum += img_value * filter_value;
}
}
outputChannel[idy * numCols + idx] = sum;
}
3.Combine blured R, G, B channels back to RGB.
__global__
void recombineChannels(const unsigned char* const redChannel,
const unsigned char* const greenChannel,
const unsigned char* const blueChannel,
uchar4* const outputImageRGBA,
int numRows,
int numCols)
{
const int2 thread_2D_pos = make_int2( blockIdx.x * blockDim.x + threadIdx.x,
blockIdx.y * blockDim.y + threadIdx.y);
const int thread_1D_pos = thread_2D_pos.y * numCols + thread_2D_pos.x;
if (thread_2D_pos.x >= numCols || thread_2D_pos.y >= numRows)
{
return;
}
unsigned char red = redChannel[thread_1D_pos];
unsigned char green = greenChannel[thread_1D_pos];
unsigned char blue = blueChannel[thread_1D_pos];
//Alpha should be 255 for no transparency
uchar4 outputPixel = make_uchar4(red, green, blue, 255);
outputImageRGBA[thread_1D_pos] = outputPixel;
}
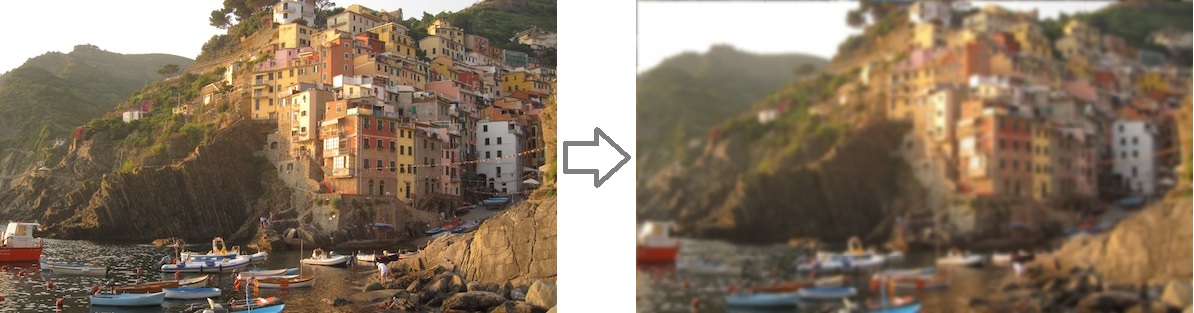
CPU: ~1035 msec (Intel i3-6006U 2GHz)
GPU: ~4 msec (Nvidia 920MX)
Github link.
Ideas to try:
- Load the image patch and the filter’s weights into the shared memory.
Problem set #3: HDR Tone-mapping
The main task for this assignment is to implement efficien Exclusive scan algorithm.
Steps to follow:
- Find the minimum and maximum value in the input data (extracted from an RGB image the logLuminance = log(Luma) channel). Perform min/max reduction.
- Subtract min and max values to find the range.
- Generate a histogram of all the values in the logLuminance channel using the formula: bin = (lum[i] - lumMin) / lumRange * numBins
- Perform an exclusive scan (prefix sum) on the histogram to get the cumulative distribution of luminance values.
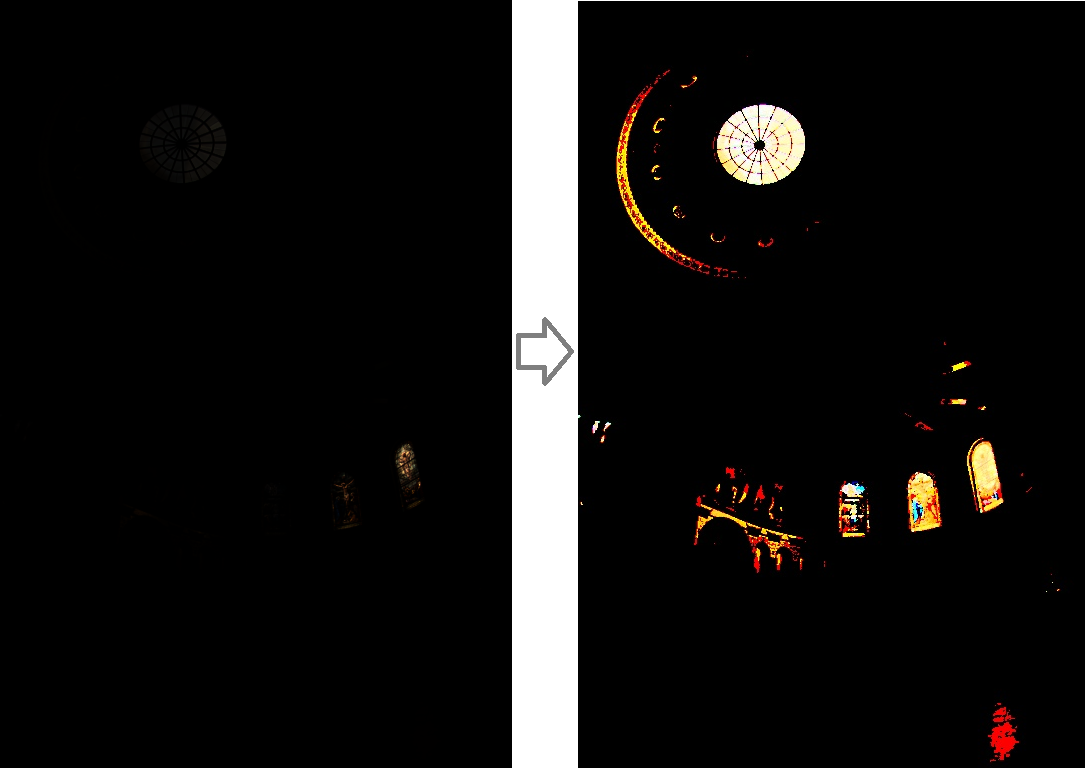
CPU: ~9 msec (Intel i3-6006U 2GHz)
GPU: ~2-4 msec (Nvidia 920MX)
Github link.
Ideas to try:
- Use shared memory for calculating a histogram for a thread block then copy the result to the global memory.
Problem set #4: Red Eye Removal
Main task for this assignment is to implement Radix sorting algorithm.
For each bit repeat next steps:
- Calculate the histogram of the number of occurrences of 0s and 1s.
- Calculate predicates (whether a particular bit is 0 or 1), perform Exclusive-scan on it (block based), save the last value of the prefix sum in the block to use it later to calculate the correct offset moving array’s elements.
- For each thread block (as in the step 2) apply corresponding offsets to the calculated prefix sum.
- Move elements in the Value and Position arrays. Output indexes for elements which predicates are 0s match with the calculated prefix sum, if predicate is 1 - add the histogram[0] value to the corresponding prefix sum value.
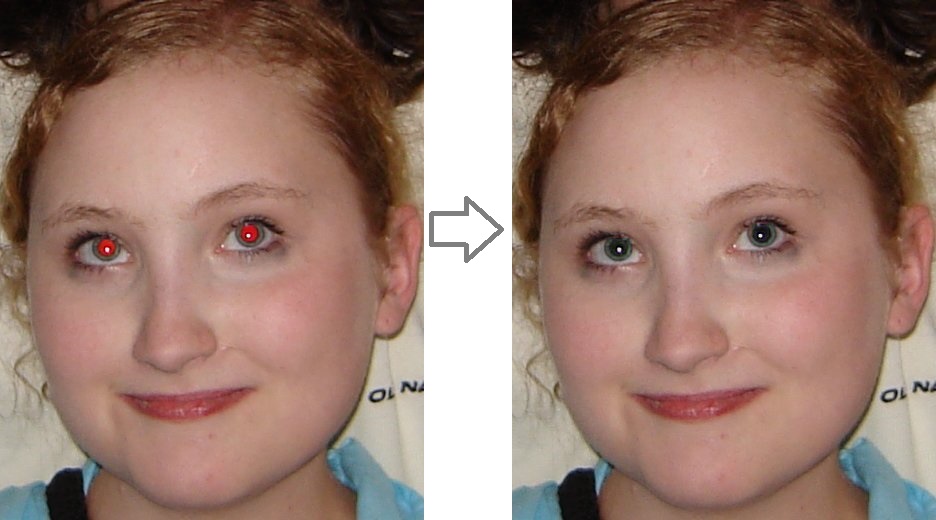
CPU: ~120 msec (Intel i3-6006U 2GHz)
GPU: ~72 msec (Nvidia 920MX)
Github link.
Ideas to try:
- get rid of the step #3 by changing the Exclusive-sum algorithm.
For each bit repeat next steps:
- Calculate the histogram of the number of occurrences of 0s and 1s.
- Calculate predicates (whether a particular bit is 0 or 1) for each value.
- Apply Exclusive scan from thrust library.
- Move elements in the Value and Position arrays. Output indexes for elements which predicates are 0s match with the calculated prefix sum, if predicate is 1 - add the histogram[0] value to the corresponding prefix sum value.
GPU: ~50 msec (Nvidia 920MX)